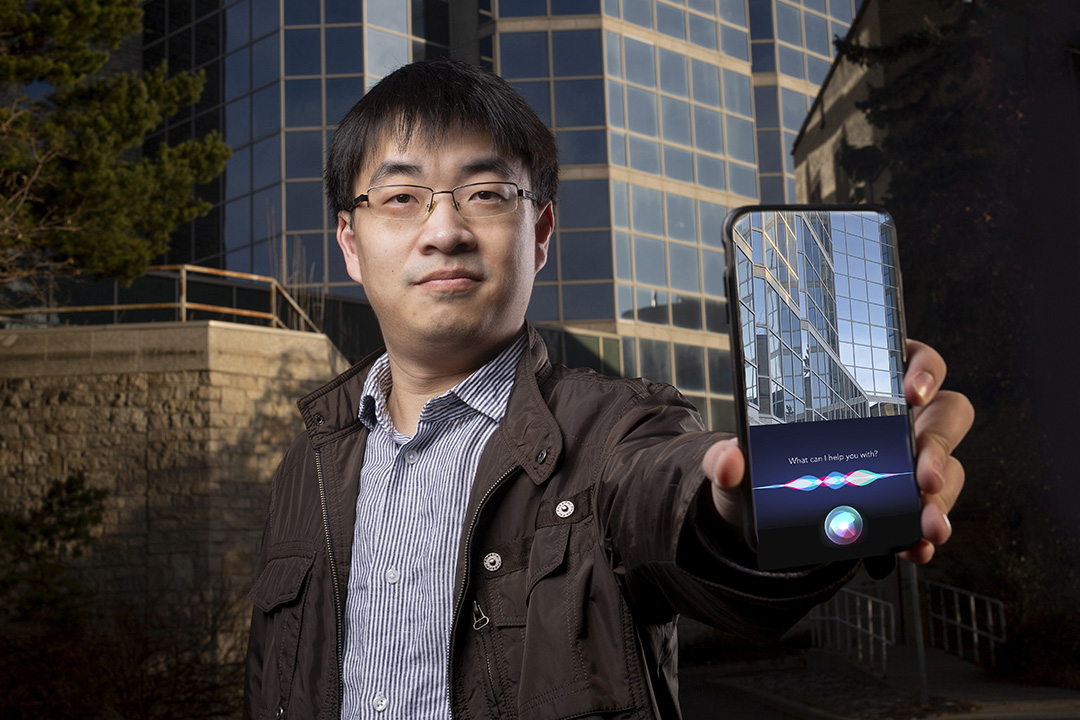
New USask artificial intelligence makes 'smart' apps faster, more efficient
This research may lead to a different way to design apps and operating systems for digital devices such as tablets, phones and computers.
By Federica GiannelliTired of Siri or Google Assistant draining your phone battery?
A new University of Saskatchewan (USask) artificial intelligence computer model holds promise for making "smart" apps such as Amazon, Apple, and Google’s virtual assistants safer, faster and more energy efficient.
"Smart" services such as facial recognition, weather forecasting, virtual assistants, and language translators rely on an artificial intelligence (AI) technology called "deep learning" to predict user patterns.
But these AI processes often require too much storage to be run locally on mobiles, so the data is sent to external servers over the Internet, which requires lots of power, drains the phone battery, and may increase a user’s privacy risk.
"My method breaks down the AI computational processes in smaller chunks and this helps run the 'smart' apps locally on the phone, rather than relying on external servers, while reducing power consumption," said Hao Zhang, a USask electrical and computer engineering post-doctoral fellow.
"This research may lead to a different way to design apps and operating systems for our digital devices such as tablets, phones and computers."
Zhang ran accurate simulations to compare his AI model with those used on current phone systems, and found that his AI model can simultaneously run multiple apps more efficiently than devices currently on the market. His model performed 20 per cent faster—twice as fast in some cases—and showed that batteries can last twice as long. The results are published in the top-tier journal IEEE Transactions on Computers.
Zhang found that AI processes can handle data efficiently using smaller four-bit sequences with variable length, so he built his model with these shorter "bit chunks." Current devices use a fixed 32-bit sequence to process data more accurately. As a result, phones or computers are not as fast and require more memory space to store the data.
"Large bit sequences are not always required to process data," said Seok-Bum Ko, a USask electrical and computer engineering professor and Zhang’s supervisor. "Shorter sequences can be used to save power and increase speed performance, but can still guarantee enough accuracy for the app to function."
While the results are promising, Zhang and Ko are working to integrate their AI model with larger computer and phone systems, and to test how the model will function in real-world processors.
"If all goes well with our research, we may have our model integrated with apps and systems in three or four years," said Ko.
Zhang, whose research is funded by the federal agency NSERC, decided USask was the right place to pursue a PhD degree to study deep learning. As a master's student at the City University of Hong Kong, he had a life-changing experience during his exchange internship at USask under Ko's supervision.
"The two universities have a good research collaboration and the internship experience here was great," he said. "We have good research equipment at USask that can support me to do a lot of experiments and to study many research topics, especially deep learning."
Because of his work on AI, Zhang was awarded the 2020 Madan and Suman Gupta Award for best engineering PhD dissertation by USask. USask PhD graduate Dongdong Chen, Ko's former student, also collaborated to advance Zhang’s project.
Federica Giannelli is a CGPS-sponsored graduate student intern in the USask research profile and impact unit.
This article first ran as part of the 2020 Young Innovators series, an initiative of the USask Research Profile and Impact office in partnership with the Saskatoon StarPhoenix.